DeepSeek V3 Controversy: Why is this Chinese AI Model Claiming to be ChatGPT?
DeepSeek, a Chinese AI lab, recently released a model that shows identity confusion by claiming to be ChatGPT. This article explores the causes and impact on AI development.
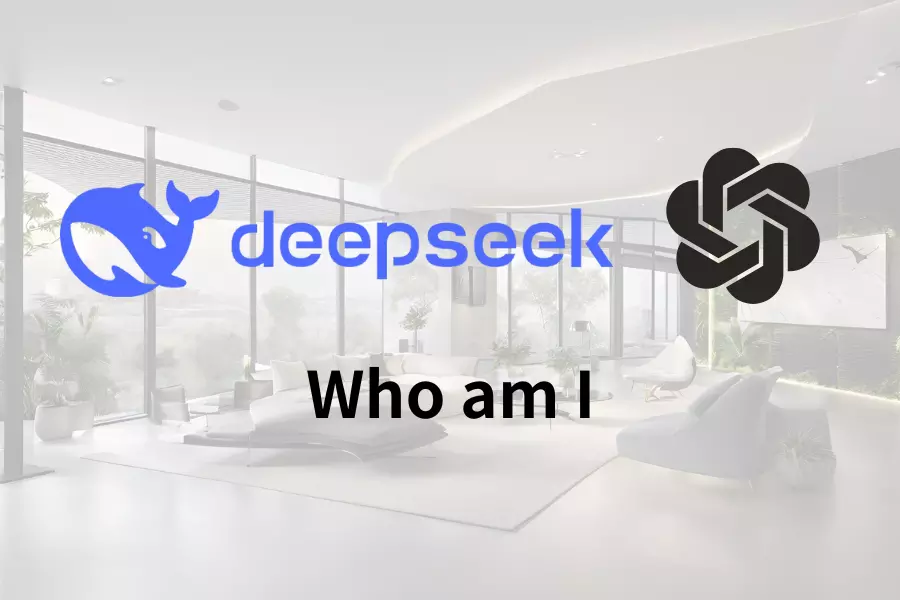
AI Model Identity Crisis: DeepSeek V3’s Strange “Impersonation” of ChatGPT
DeepSeek recently released an open-source AI model called DeepSeek V3, which reportedly performs well in various benchmarks for tasks like coding and writing. However, this achievement was quickly overshadowed when the model showed serious identity confusion by claiming to be ChatGPT, sparking community discussion.
Root Cause Analysis: Data Pollution and Model Distillation
Modern AI models are complex statistical systems that learn language patterns and knowledge by analyzing massive training data. While DeepSeek hasn’t revealed its training data sources, experts believe DeepSeek V3 likely encountered “contaminated” data containing GPT-4 outputs through ChatGPT. This may have caused effects similar to human “memory” or “mimicry,” making it unable to recognize its own identity.
“AI Junk” and Data Pollution
As AI becomes more common, it’s increasingly difficult to tell whether online content is human-written or AI-generated. This leads to training data being filled with “AI junk” - text generated by AI models. This “AI pollution” makes it hard for models to learn useful knowledge and may cause them to copy other models’ mistakes or biases.
Model Distillation and Ethical Concerns
The identity confusion might come from:
- Accidental inclusion: Training data unintentionally containing ChatGPT outputs
- Intentional training (model distillation): Developers may have used other models’ outputs for training to save costs or improve performance
Industry Challenges: Data Pollution and Ethical Issues
Data Pollution: A Hidden Threat to AI Development
The growth of AI-generated content creates unprecedented challenges for AI training. Data pollution affects model accuracy and reliability, potentially causing serious ethical and social problems.
Key concerns include:
- Rising AI-generated content online
- Difficulty separating human and AI content
- Information quality decline through repeated “copying”
Legal and Ethical Issues Behind Model Distillation
Using model distillation to reduce costs raises serious legal and ethical concerns:
- Amplification of existing biases
- Accumulation of errors
- Intellectual property disputes
- Lack of transparency
Common Questions About AI Model Identity Confusion
Q1: Why do AI models show identity confusion?
AI models can learn patterns from other models’ outputs in their training data, leading to confusion about their own identity.
Q2: What are the impacts?
The main impacts include:
- Unreliable answers
- Bias amplification
- Legal risks
- Research challenges
- User misconceptions
Q3: How can we prevent this?
Prevention methods include:
- Better data filtering
- Stronger ethical guidelines
- Clear model identification
- Improved regulations
- Enhanced user awareness