Meta Motivo: A Breakthrough AI Full-Body Humanoid Control Model | Full Analysis and Applications
Summary
Meta’s latest breakthrough AI model, Motivo, uses innovative unsupervised reinforcement learning algorithms to achieve full-body motion control of virtual humanoid agents. This technology can perform diverse tasks without additional training, revolutionizing the metaverse and virtual reality experiences. Motivo not only provides virtual avatars with more natural and fluid movements but also opens up new possibilities for immersive interactions.
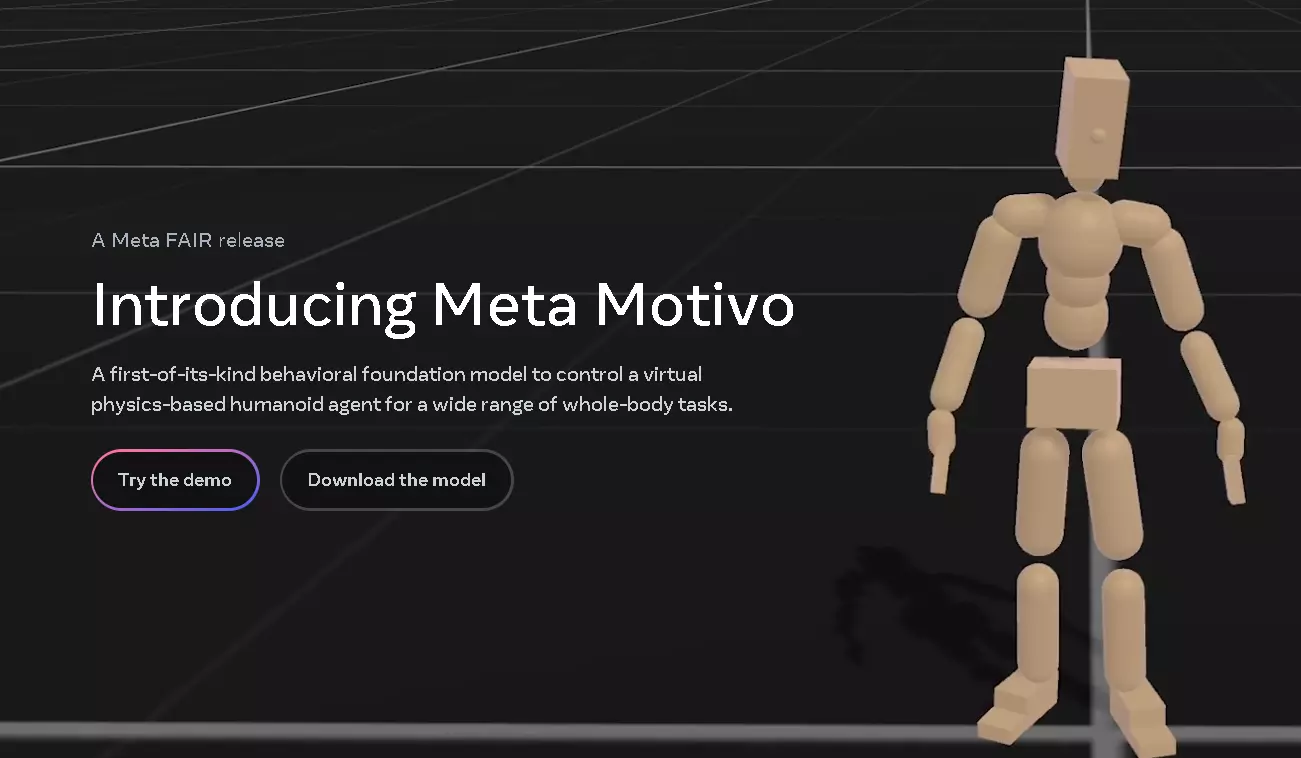
Image captured from: https://metamotivo.metademolab.com/
Motivo’s core advantage lies in its powerful generalization capabilities and realistic simulation of the physical world, enabling it to exhibit natural and diverse behaviors in virtual environments. Here are its main features:
1. Powerful Generalization Without Additional Training (Zero-Shot Learning)
Motivo’s most notable feature is its exceptional zero-shot learning capability. This means Motivo can perform various complex actions and behaviors without needing additional training for specific tasks, demonstrating high adaptability. Specifically, Motivo can:
- Accurately Track and Mimic Actions: It can capture and mimic human or other biological movements, achieving realistic behavior replication.
- Flexibly Achieve Specific Poses: It can quickly and stably achieve various specific poses based on commands or environmental changes, such as standing, sitting, or raising hands.
- Efficiently Optimize Reward Goals: It can autonomously learn and optimize strategies to achieve goals within given environments and objectives, showcasing intelligent behavior decision-making.
2. Physically-Based Realistic Simulation
Motivo deeply integrates a physics engine, ensuring all movements adhere to real-world physical laws, significantly enhancing the realism and immersion of virtual environments. This allows Motivo to:
- Accurately Simulate Gravity Effects: It accurately simulates the impact of gravity on objects and characters, making movements more natural and compliant with physical laws.
- Precisely Calculate Joint Limits: It considers the range and limits of biological joints, avoiding unnatural movements or clipping.
- Naturally Handle Environmental Interactions: It can realistically simulate interactions between characters and the environment, such as collisions, friction, and support, making the virtual world more interactive.
- Effectively Handle External Disturbances: Even under external disturbances, it can maintain balance and stable movements, demonstrating high robustness.
Innovative Technical Architecture
Motivo’s outstanding performance stems from its innovative technical architecture, with the core being the FB-CPR algorithm and a meticulously designed network structure. Here’s a detailed explanation:
1. FB-CPR Algorithm: The Core Engine Driving Motivo
The Forward-Backward representations with Conditional Policy Regularization (FB-CPR) algorithm is the core of Motivo, combining the advantages of unsupervised learning and imitation learning, granting Motivo powerful generalization and realism. Key features of FB-CPR include:
- Combining Unsupervised Forward-Backward Representation Learning: FB-CPR analyzes the forward and backward relationships in action sequences to learn the internal structure and patterns of movements, effectively extracting useful feature representations without manually labeled data. This allows Motivo to learn from large amounts of unlabeled data, significantly enhancing model generalization.
- Constraining Policy Behavior Through Imitation Learning: FB-CPR uses imitation learning to constrain policy behavior, making it closer to natural human or biological movements. This helps avoid unnatural movements or behaviors, enhancing the realism of virtual avatars.
- Achieving Unified Representation of States, Actions, and Rewards: FB-CPR embeds states, actions, and rewards into the same latent space, enabling the model to more effectively learn their relationships and make smarter decisions. This unified representation is key to Motivo’s ability to perform diverse tasks.
2. Sophisticated Network Architecture: Efficiently Executing the FB-CPR Algorithm
To efficiently execute the FB-CPR algorithm, Motivo adopts a sophisticated network architecture, including the following key components:
- Embedding Network: The embedding network processes the agent’s state information, such as joint angles, velocities, and positions, converting it into high-dimensional embedding vectors. These vectors capture the agent’s current state, providing crucial input for the policy network.
- Policy Network: The policy network outputs the agent’s next action commands based on the vectors generated by the embedding network. The policy network is designed to learn the strategies constrained by the FB-CPR algorithm, enabling the agent to perform movements that are natural and compliant with physical laws.
Experimental Results and Evaluation
To validate Motivo’s performance and generalization capabilities, rigorous quantitative and qualitative evaluations were conducted, comparing it with other advanced models. The results show that Motivo excels in multiple aspects.
1. Quantitative Evaluation: Demonstrating Superior Generalization
Motivo was evaluated on multiple standard benchmarks, with results showing:
- Achieving 61%-88% of specialized model performance across multiple tasks: This indicates that Motivo can reach high performance levels of specialized models without being trained for specific tasks, fully demonstrating its powerful generalization capabilities. This achievement is particularly significant as it proves Motivo can be effectively applied to various virtual environments and tasks without requiring extensive time and resources for additional training.
- Outstanding performance in action tracking tasks, only behind Goal-TD3: In the critical task of action tracking, Motivo’s performance was only surpassed by the Goal-TD3 model, specifically optimized for this task. This further proves Motivo’s excellence in mimicking and tracking complex movements.
- Demonstrating excellent cross-task generalization: Overall, Motivo showed the ability to effectively perform across various tasks, proving its excellent cross-task generalization capabilities, making it more flexible and valuable in practical applications.
2. Qualitative Evaluation: Movements More Natural and Closer to Human
In addition to quantitative evaluation, Motivo underwent human evaluation to more intuitively understand whether the movements it generates are natural and realistic. The evaluation results showed:
- Generating more natural movements compared to single-task TD3: Human evaluators generally found that Motivo’s movements were more natural, fluid, and aligned with human movement habits than those generated by the TD3 model designed for a single task.
- Achieving a better balance between performance and behavior quality: Motivo not only excelled in performance but also considered behavior quality, avoiding unnatural movements in pursuit of high scores. This indicates that Motivo can exhibit more human-like behaviors in virtual environments.
- Demonstrating movement characteristics closer to humans: Observing Motivo’s movements, one can see that its movement trajectories, speed changes, and limb coordination are closer to real human movement characteristics, enhancing immersion in virtual environments.
Application Prospects: The Infinite Possibilities of Motivo
Motivo’s breakthrough technology not only holds significant academic importance but also shows broad application prospects across multiple fields, promising to revolutionize life with Motivo.
Motivo’s natural motion control capabilities will significantly enhance the realism and immersion of metaverse and virtual reality experiences:
- Enhancing the Realism of Virtual Characters: Motivo gives virtual characters more natural and fluid movements, making them more like real humans in the virtual world, greatly enhancing user immersion. For example, virtual avatars can walk, run, jump, interact with other virtual characters, and even make more subtle facial expressions and body language.
- Enhancing User Interaction Experience: More natural virtual character movements will make interactions in the virtual world more intuitive and natural. For example, users can interact more naturally with objects or characters in the virtual environment through gestures or body movements, such as picking up items or shaking hands with other users.
- Supporting Complex Virtual Scene Construction: Motivo can handle complex environmental interactions, making it possible to build more complex and realistic virtual scenes. For example, virtual characters can walk, climb, and jump on complex terrains, even maintaining balance under external disturbances, greatly expanding the possibilities of virtual worlds.
2. Robotics Control: Accelerating the Development of Humanoid Robots
Motivo’s technology also brings new possibilities to the field of robotics control:
- Assisting in Humanoid Robot Development: Motivo’s motion control algorithms can be applied to the development of humanoid robots, helping them achieve more natural and flexible movements. This will accelerate the research and development of humanoid robots and reduce development costs.
- Optimizing Motion Planning Algorithms: Motivo’s unsupervised learning methods can help robots autonomously learn more optimized motion planning strategies, enabling them to better adapt to different environments and tasks. For example, robots can learn how to walk on complex terrains, navigate crowded environments, and perform precise operational tasks.
- Enhancing Robot Mobility: Motivo’s physics engine integration technology can help robots more accurately simulate real-world physical laws, enhancing their mobility and stability. For example, robots can maintain balance under external disturbances or more precisely control their limbs during complex movements.
3. Computer Animation and Gaming: Creating More Vibrant Virtual Worlds
Motivo’s technology can also be applied to the computer animation and gaming industries:
- Improving NPC Behavior Generation: Motivo can be used to generate more natural and realistic NPC behaviors, enhancing the immersion and challenge of games. For example, NPCs can respond more intelligently to the environment and player actions, such as fleeing, hiding, or attacking.
- Optimizing Character Animation Production: Motivo can help animators produce character animations more quickly and efficiently, reducing the workload of manual adjustments. For example, animators can use Motivo to generate initial animation skeletons and then make fine adjustments.
- Enhancing Game Interaction Realism: More natural NPC behaviors and more realistic character animations will significantly enhance the realism of game interactions, allowing players to immerse themselves more deeply in the game world.
Frequently Asked Questions
To help everyone better understand Meta Motivo, we have compiled some common questions and provided answers:
A: The biggest difference between Meta Motivo and traditional motion control models lies in its powerful generalization capabilities. Traditional models typically require extensive training for specific tasks to achieve good results. In contrast, Motivo uses a zero-shot learning approach, meaning it can handle various types of tasks within the same framework without needing additional or retraining for new tasks, such as action tracking, pose achievement, and reward goal optimization. This no-additional-training feature significantly reduces development costs and time and increases model flexibility and applicability. Additionally, Motivo deeply integrates a physics engine, producing more natural and physically compliant movements, which is challenging for traditional models.
Q2: What are the main limitations of Motivo currently?
A: Although Motivo excels in many aspects, it still has some limitations:
- Relatively poor performance when handling fast movements and ground interactions: Motivo performs relatively weaker when handling movements that require extremely quick responses or complex interactions with the ground, such as fast running, jumping, or rolling, and there is room for improvement.
- Occasional unnatural jittering: In some cases, Motivo’s generated movements may exhibit slight unnatural jittering, possibly due to the model not fully mastering certain subtle movement details during the learning process.
Efforts are being made to research and improve these issues to further enhance Motivo’s performance and stability.
Q3: When can this technology be applied to real products?
A: Meta has released model code and benchmark datasets for Motivo, meaning developers can start exploring and applying it to various real products and projects now. The developer community is encouraged to actively participate and jointly drive the development and application of this technology. In the future, Motivo will continue to be updated and improved, and more potential application scenarios will be explored, such as the metaverse, virtual reality, robotics control, computer animation, and gaming.
Conclusion: Motivo Leads a New Era in AI Control Technology
The advent of Meta Motivo not only represents a significant breakthrough in the field of AI control but also heralds the dawn of a new era. Its unique zero-shot learning capabilities endow virtual avatars and robots with unprecedented flexibility and adaptability, opening up infinite possibilities in fields such as the metaverse, robotics technology, computer animation, and gaming.
Motivo’s core advantages are:
- Powerful Generalization Without Additional Training: Motivo can perform various complex actions and behaviors without specific task training, significantly reducing development costs and time and increasing application flexibility.
- Physically-Based Realistic Simulation: Motivo deeply integrates a physics engine, ensuring all movements comply with real-world physical laws, significantly enhancing the realism and immersion of virtual environments.
- More Natural Movements Closer to Humans: Through human evaluation, Motivo demonstrates more natural and fluid movements than traditional models, better aligning with human movement habits.
These advantages position Motivo for broad application prospects in multiple fields, such as:
- Metaverse and Virtual Reality: Creating more realistic virtual characters, enhancing user interaction experiences, and supporting more complex virtual scene construction.
- Robotics Control: Assisting in humanoid robot development, optimizing motion planning algorithms, and enhancing robot mobility.
- Computer Animation and Gaming: Improving NPC behavior generation, optimizing character animation production, and enhancing game interaction realism.
Admittedly, Motivo currently has some limitations, such as relatively poor performance when handling fast movements and ground interactions, and occasional unnatural jittering. However, Meta has released Motivo’s model code and benchmark datasets, not only showcasing Meta’s open attitude towards technological development but also meaning that developers worldwide can participate in improving and applying Motivo, jointly driving the development of this technology.
Looking to the future, with continuous technological advancements and community efforts, Motivo will overcome current limitations and demonstrate its powerful potential in more fields, bringing richer, more convenient, and more enjoyable experiences to life with Motivo. The open-source nature of Motivo will also accelerate the development of related fields.