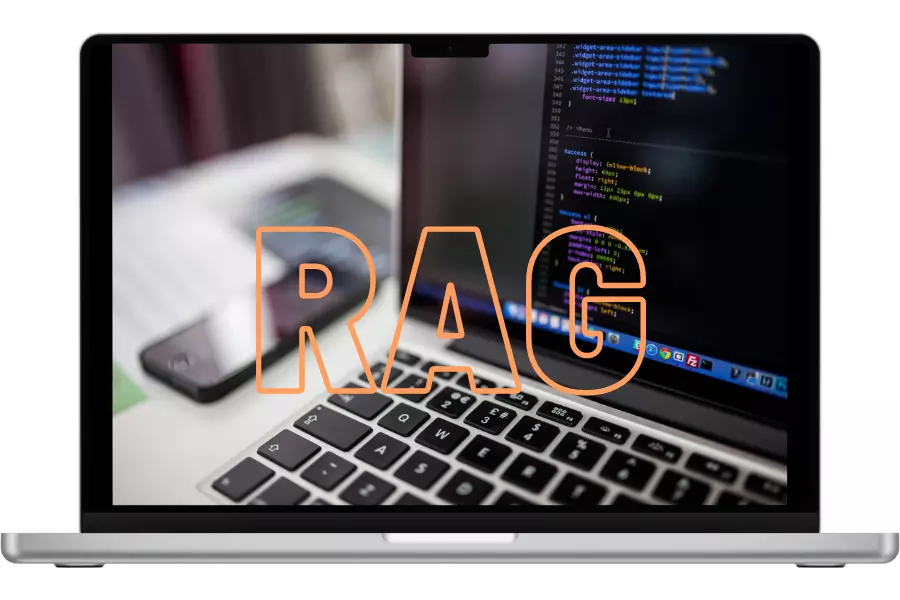
RAG-as-a-Service: Unleashing the Potential of Generative AI for Enterprises
With the rise of Large Language Models (LLMs) and generative AI trends, integrating generative AI solutions into enterprises can significantly boost productivity. If you’re new to generative AI, the plethora of terminology might seem daunting. This article will explain the basic terms of generative AI and guide you on how to customize AI solutions for your business through RAG-as-a-Service.
What is Retrieval-Augmented Generation (RAG)?
Retrieval-Augmented Generation (RAG) is a key concept for implementing LLMs or generative AI in enterprise workflows. RAG leverages pre-trained Transformer models to answer business-related questions by injecting relevant data from your specific knowledge base during the query process. This data is untrained on by the LLMs, resulting in accurate and pertinent responses.
RAG is both cost-effective and efficient, making generative AI more accessible. Let’s explore some key terms related to RAG.
Key Terms of RAG
Chunking
LLMs are resource-intensive and are trained using manageable data lengths known as “context windows.” The context window varies depending on the LLM used. To address its limitations, business data, such as documents or text literature, is divided into smaller chunks. These chunks are used during the query retrieval process.
Since these chunks are unstructured and queries might differ syntactically from knowledge base data, semantic search is used to retrieve these chunks.
Vector Databases
Vector databases like Pinecone, Chromadb, and FAISS store embeddings of business data. Embeddings convert text data into numerical forms stored in high-dimensional vector spaces where semantically similar data points are located close to each other.
When a user queries, the query’s embedding is used to find semantically similar chunks in the vector database.
RAG-as-a-Service (RaaS) Process
Implementing RAG for your enterprise can be daunting if you lack technical expertise. This is where RAG-as-a-Service (RaaS) comes into play.
Frequently Asked Questions
1. What is RAG-as-a-Service (RaaS)?
RAG-as-a-Service (RaaS) is a comprehensive solution that handles the entire retrieval-augmented generation process for your enterprise. This includes data chunking, storing embeddings in vector databases, and managing semantic search to retrieve relevant data for queries.
2. How does chunking help in the RAG process?
Chunking breaks down large business documents into smaller chunks that fit the LLM’s context window. This division allows the LLM to process and retrieve relevant information more efficiently using semantic search.
3. What are vector databases and why are they important?
Vector databases store numerical representations (embeddings) of business data. These embeddings enable efficient retrieval of semantically similar data during queries, ensuring the LLM provides accurate and relevant responses.
4. How to set up RAG
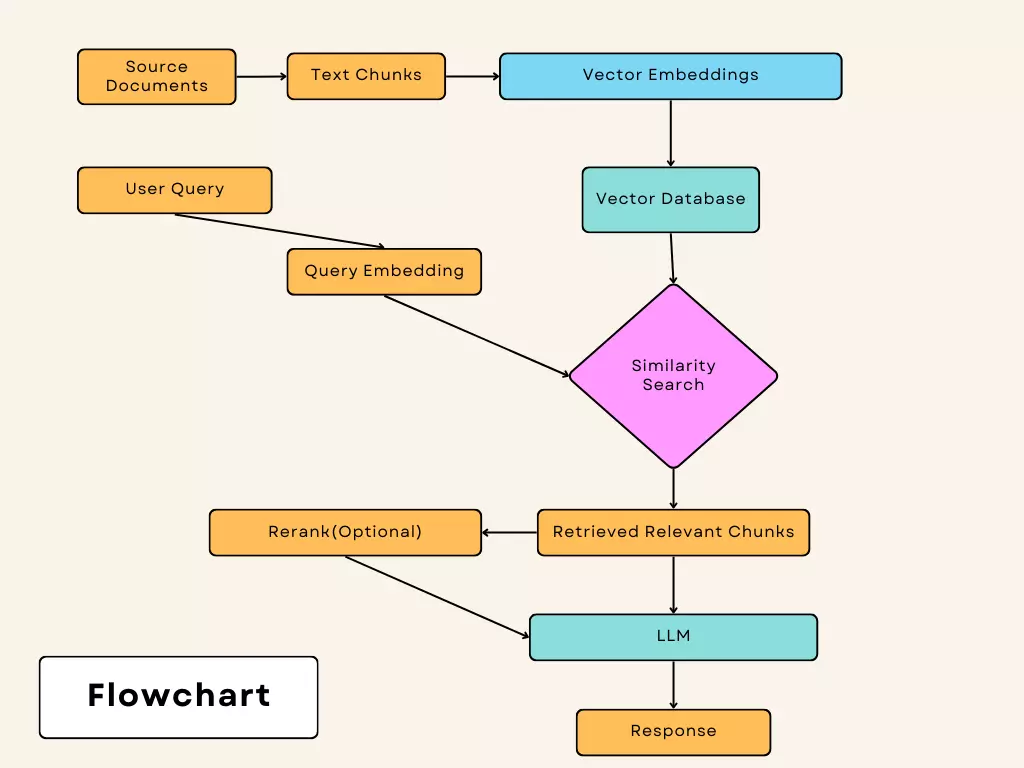